Machine Learning Malware Analysis: A Revolution in Cybersecurity
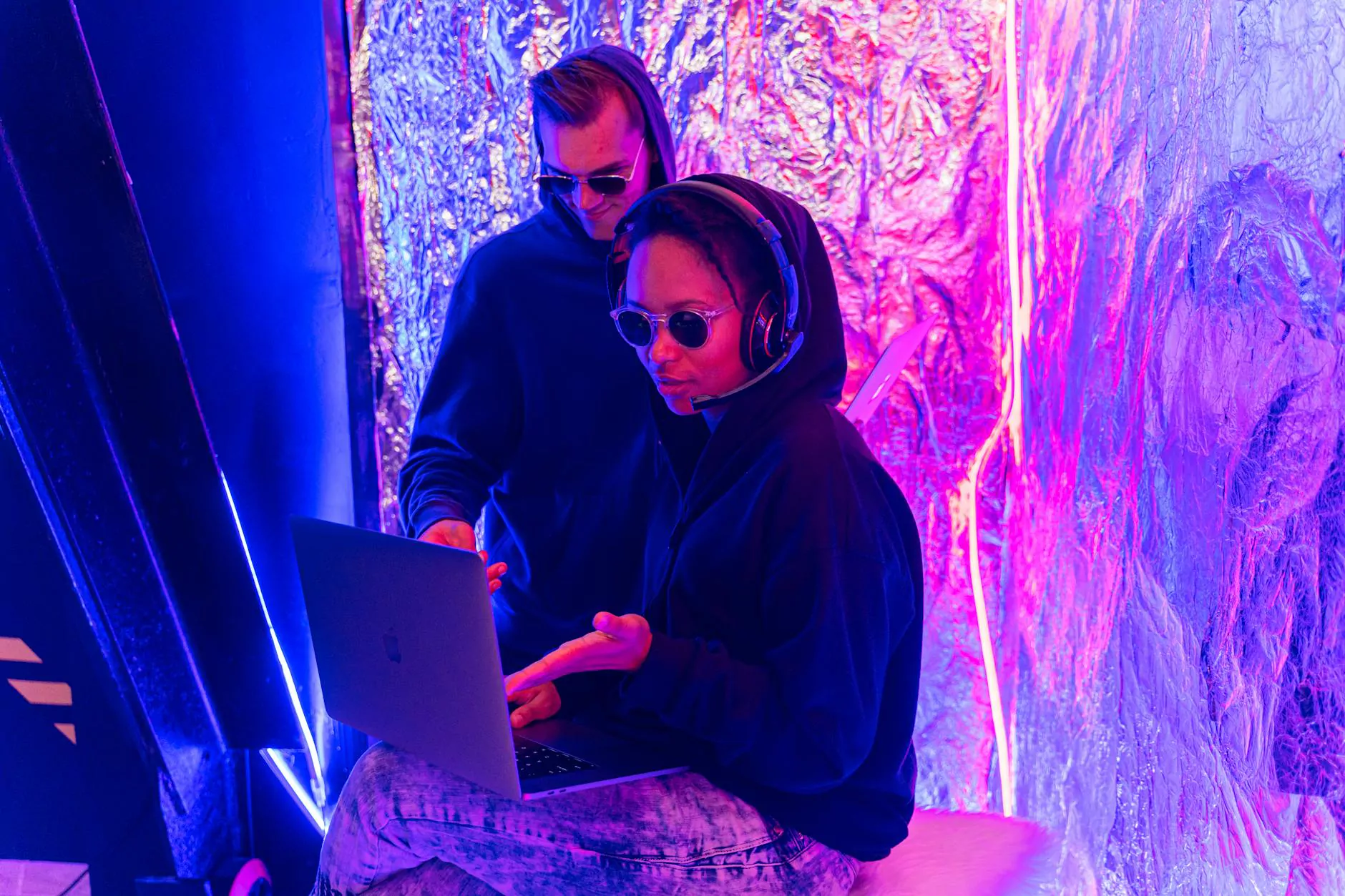
In today's digital landscape, the rise in cyber threats has made it crucial for businesses to adopt proactive measures in order to protect their data and integrity. The advent of machine learning malware analysis represents a significant leap in cybersecurity capabilities, enabling organizations to react swiftly and efficiently to emerging threats. This comprehensive article will explore the intricate relationship between machine learning technologies and malware analysis, highlighting its implications for IT Services & Computer Repair and Security Systems.
The Emergence of Machine Learning in Cybersecurity
Machine learning, a subset of artificial intelligence, has transformed various industries over the years, and cybersecurity is no exception. Traditional methods of malware detection utilize signature-based approaches that can be effective but are often unable to identify new or evolving threats. In contrast, machine learning algorithms can analyze vast amounts of data, recognize patterns, and detect anomalies, making them ideally suited for the dynamic nature of malware threats.
Understanding Malware and Its Impact on Businesses
Before delving into machine learning malware analysis, it is essential to understand what malware is and its impact on businesses. Malware—short for malicious software—includes various types of harmful software, such as viruses, worms, trojans, ransomware, and spyware. The consequences of malware infections can be devastating, including:
- Financial Loss: Businesses can incur significant costs from system repairs, data recovery, and regulatory fines.
- Data Breaches: Compromised data can lead to identity theft and loss of customer trust.
- Operational Disruption: Malware can halt business operations, affecting productivity and service delivery.
- Reputation Damage: A successful cyberattack can tarnish a company's reputation, leading to prolonged recovery periods.
The Role of Machine Learning in Malware Detection
Machine learning aids in malware detection through several techniques. By leveraging algorithms designed to learn from data, organizations can improve their defenses against malware attacks. The following are some core functionalities of machine learning in malware analysis:
1. Pattern Recognition
Machine learning algorithms can analyze historical malware attack data to identify and learn patterns. This capability allows them to recognize potential malware behavior even when facing unfamiliar threats.
2. Behavioral Analysis
Unlike traditional signature-based detection, which relies on known malware signatures, machine learning can assess the behavior of applications. By examining how software interacts with system resources, these algorithms can flag suspicious behavior indicative of malware activity.
3. Real-time Threat Detection
Machine learning enables real-time analysis of incoming data traffic and application behavior, allowing for instant detection and response to threats. This capability drastically reduces the time spent in malware remediation.
4. Continuous Learning
Machine learning algorithms can adapt and improve based on new data. As they encounter new malware attempts, they refine their detection methods, creating a robust defense mechanism that evolves alongside cyber threats.
Implementing Machine Learning Malware Analysis
For organizations looking to implement machine learning malware analysis, several steps should be considered:
1. Data Collection
Gathering large datasets of historical malware behavior is paramount. This includes data from previous infections, attacker tactics, and network traffic.
2. Choosing the Right Tools and Frameworks
There are numerous machine learning frameworks available, such as TensorFlow, PyTorch, and Scikit-learn. Choosing the right tool depends on the specific needs of the organization and the expertise of its IT staff.
3. Training Machine Learning Models
Once the data is collected and the tool has been chosen, training machine learning models on this data is crucial. This involves feeding the models historical data and allowing them to learn the associated patterns.
4. Testing and Validation
It is essential to rigorously test and validate the models to ensure they accurately detect malware without generating excessive false positives.
5. Deployment and Ongoing Monitoring
After thorough testing, deploying the models into production and continuously monitoring their performance is essential. Ongoing updates and retraining based on new data help maintain efficacy.
Benefits of Machine Learning Malware Analysis
The integration of machine learning into malware analysis provides several advantages:
- Improved Detection Rates: Machine learning can uncover hidden malware patterns and behaviors that traditional methods may miss.
- Reduced Response Time: Automated detection and response systems enable quicker incident handling.
- Less Reliance on Human Intervention: While human analysts are invaluable, machine learning can handle a significant portion of detection and analysis autonomously.
- Scalability: Machine learning models can process vast amounts of data, making them scalable to accommodate the increasing volume of cyber threats.
Challenges and Considerations
While the advantages are numerous, organizations must also be aware of challenges associated with machine learning malware analysis:
1. Data Quality
The effectiveness of machine learning algorithms fundamentally relies on the quality of the data used for training. Poor quality data can lead to inaccurate predictions.
2. Complexity of Models
Machine learning models can be complex and require specialized knowledge for proper implementation and tuning. Organizations may need to invest in training or hiring experts.
3. Evolving Threat Landscape
The rapid evolution of cyber threats means that organizations must continuously update their models and training datasets. Failure to do so can render the analysis ineffective.
Future Trends in Machine Learning Malware Analysis
As technology continues to evolve, the role of machine learning in malware analysis is set to expand even further. Some notable future trends include:
1. Increased Integration with Threat Intelligence
Combining machine learning malware analysis with external threat intelligence sources will lead to a more comprehensive understanding of the threat landscape.
2. Collaboration between AI and Human Analysts
While machine learning can automate several processes, the human element will remain crucial. Future systems will likely enhance collaboration between AI tools and human analysts to improve overall effectiveness.
3. Ethical and Regulatory Considerations
As AI technologies become more prevalent in cybersecurity, ethical considerations related to data privacy and AI accountability will gain importance.
Conclusion
The incorporation of machine learning malware analysis into the framework of IT services and security systems stands as a promising solution in the battle against cyber threats. By harnessing the power of machine learning, businesses can enhance their malware detection capabilities, ensuring that they are better equipped to defend against the evolving landscape of cyberattacks. Continuous innovation and adaptation will be key in maintaining a robust cybersecurity posture, allowing organizations to focus on their core objectives with one less worry on their minds.
For companies striving to improve their cybersecurity strategies, investing in machine learning technologies is not just an option—it is a necessity. At Spambrella, we understand the importance of protecting your business, and we are dedicated to providing you with state-of-the-art IT services and security solutions that integrate cutting-edge technologies for comprehensive defense against malware threats. Your protection starts here!